Spark
Apache Spark requires a pack chairman and a scattered accumulating system. For bunch the board, Spark maintains autonomous (neighborhood Flash pack, where you can dispatch a pack either actually or use the dispatch substance given by the present group. It is in like manner possible to run these daemons on a lone machine for testing), YARN, Apache Notices or Cabernets.
For circled limit, Flash can interface with a wide grouping, includingHadoop Distributed File System (HDFS), Guide Document Framework (Guide FS), Cassandra, Open Stack Quick, Amazon S3, Kudu, spark record framework, or a custom game plan can be executed.
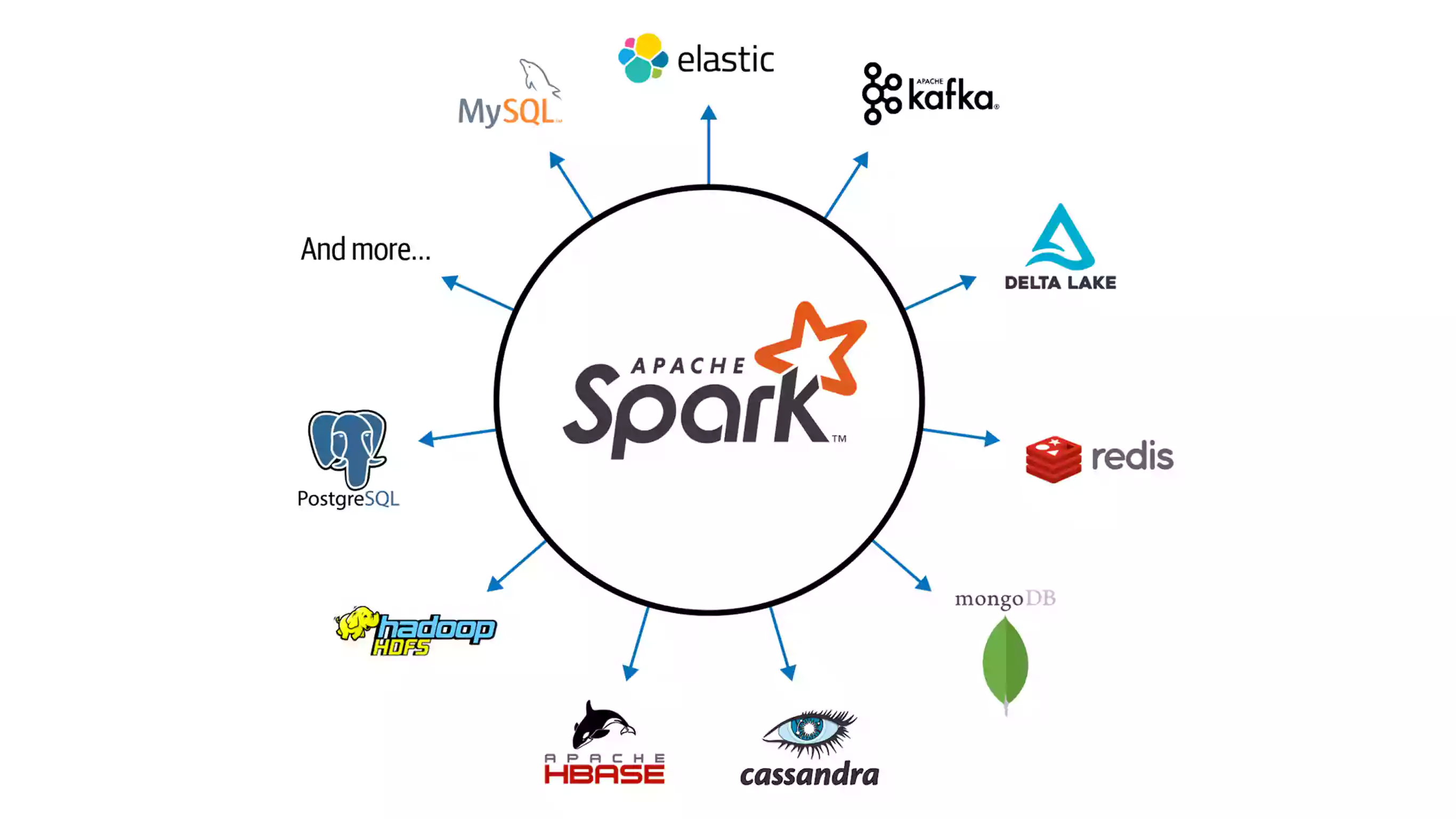
Spark moreover maintains a pseudo-passed on close by mode, commonly used particularly for headway or testing purposes, where appropriated limit isn't required and the local archive system can be used taking everything into account; in such a circumstance, Spark is run on a lone machine with one specialist for every central processor place.
Spark recommendations in excess of huge level directors that simplify it to gather equivalent applications. Additionally, you can use it instinctively from the, Python, R, and SQL shells. Spark controls a heap of libraries including SQL and b for man-made intelligence, Chart, and Flash Streaming. You can join these libraries impeccably in a comparable application.
This interface mirrors a functional/higher-demand model of programming: a "driver" program calls equivalent exercises like guide, channel or reduce on a RDD by passing an ability to Start, which by then plans the limit's execution in equivalent on the bunch. These undertakings, and additional ones like joins, acknowledge RDDs as data and produce new RDDs. RDDs are unchanging and their assignments are lazy; variation to inward disappointment is cultivated by observing the "heredity" of each RDD (the game plan of exercises that made it) so it will in general be reproduced by virtue of data incident. RDDs can contain any sort of Python, .NET, Java, or items.